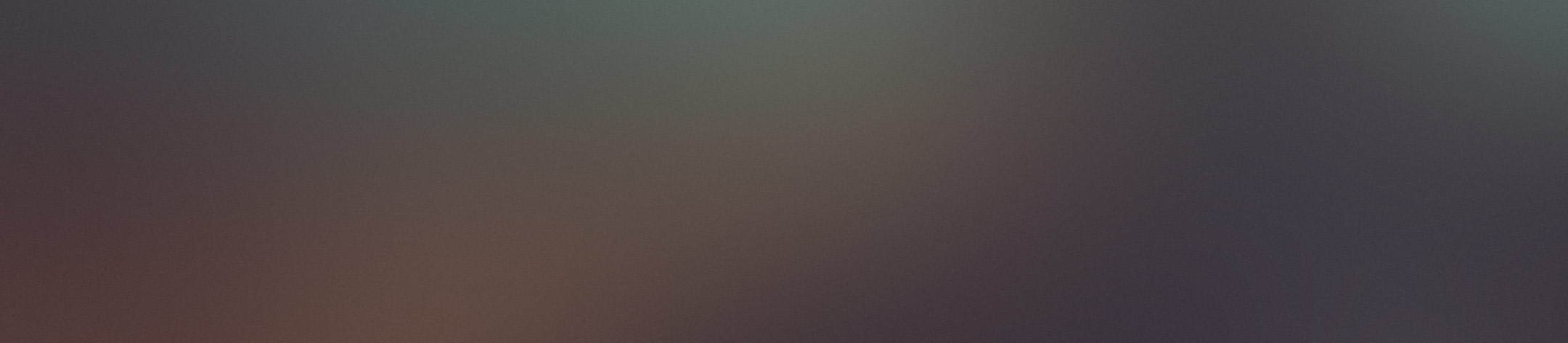
Hongwu Jiang (姜泓吾)
Assistant Professor
Microelectronics, Function Hub, HKUST(GZ)
Email: hongwujiang@hkust-gz.edu.cn
I am an Assistant Professor in the Microelectronics Thrust (Function Hub) at the Hong Kong University of Science and Technology (Guangzhou). I obtained my Ph.D. degree from Georgia Institute of Technology in 2022, where I was advised by Prof. Shimeng Yu. My research interests are in circuit and architecture design for efficient computing systems with CMOS and beyond CMOS technologies. To date, I have published more than 20 papers in leading conferences (VLSI, DAC, ISSCC, etc.) and journals (IEEE TCAS-I, IEEE T-Computer, JSSC, TCAD, etc.).
Openings
I’m looking for self-motivated Ph.D. students (Fall 2025) , and postdoctoral scholars to join our lab at MICS, Function Hub, HKUST(GZ). We also offer RA positions. Please email me your resume and other support material if you are interested.
Work Experience
- Assistant Professor
HKUST, GZ. Start from Jan, 2023 - Research Intern
IMEC, US. Summer 2021
Education
-
Ph.D. in Electrical and Computer Engineering
advised by Prof. Shimeng Yu
Georgia Institute of Technology, 2019 -- 2022 -
M.S. in Electrical Engineering
Arizona State University, 2012 -- 2014 -
B.S. in Automation
Dalian University of Technology, 2008 -- 2012